Improved drought prediction using wavelet analysis
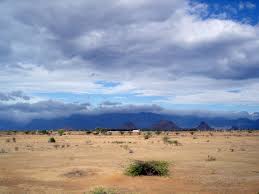
Drought models provide practical tools for managers to forecast drought indices which can quantify various aspects of a drought. A new study by Dr Ravinesh Deo and his colleagues shows that wavelet pre-processing of predictor data sets can enhance drought forecasting accuracy.
The researchers developed a wavelet-hybrid extreme learning machine (W-ELM) model, for three stations in Australia, to address issues related to non-stationarity, periodicity and stochasticity of drought model input data.
According to the researchers, predictive models have limited ability to unveil the physical structure of hydrological input data, especially for inputs that are (generally) non-linear and (potentially) non-stationary. The non-stationarity caused by trends or seasonal variation have an impact on the accuracy of models.
“Application of the wavelet transformation to extract time–frequency domain information helped capture patterns in the stochastic nature of drought model predictors.” said the authors.
The paper utilised wavelet transformation as an ancillary tool, for the first time, to feed stochastic variations, periodicity and trends in the hydrologic data using an extreme learning machine model to predict the effective drought index.
The authors noted that comparisons of W-ELM with wavelet-hybrid least square support vector regression (W-LSSVR) and neural network (W-ANN) found the W-ELM model was computationally more efficient with a faster running time and the majority of forecasting errors in low frequency bands.
According to Dr Deo, “our results have demonstrated the usefulness of W-ELM over W-ANN and W-LSSVR models and the benefits of discrete wavelet transformation of the predictor (input) data to improve the performance of a drought forecasting models”.
Article: ‘Forecasting effective drought index using a wavelet extreme learning machine (WELM) model ’, Stochastic Environmental Research and Risk Assessments, doi: 10.1007/s00477-016-1265-z