‘Extreme learning machine’ good at simulating streamflow
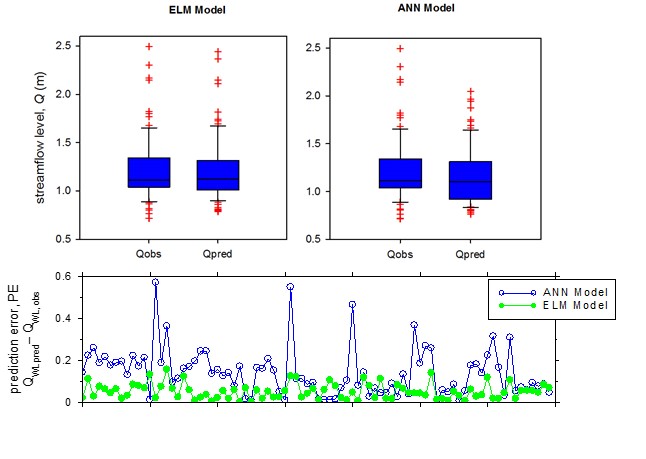
Predictive models for streamflow have practical implications for better understanding drought hydrology, eco-hydrology and environmental monitoring, agriculture and water resource management.
Recent work by Dr Ravinesh Deo and fellow researchers found that an extreme learning machine model can be used as an efficient approach for streamflow simulation and outperformed artificial neural network models on multiple measures.
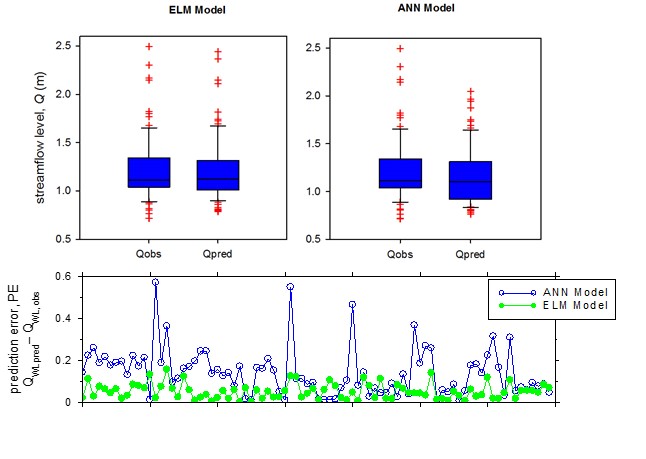
(Top) Boxplot of predicted (Qobs) and simulation streamflow (Qpred) and (Bottom) Prediction error (PE = QWL pred – QWL obs) using extreme learning machine (ELM) compared with an ANN model for Gowrie Creek.
The research employed a state-or-art extreme learning machine algorithm with rainfall, climate indices and sea surface temperatures as inputs to simulate mean streamflow water level. The analysis was applied to hydrological sites in eastern Queensland (Gowrie Creek, Albert, and Mary River) and the performance was compared to that of a traditional artificial neural network model.
According to Dr Deo, “the results found that with the optimum set of input combinations, both models provided accurate simulation performance where extreme learning machine was seen to outperform the neural network model.”
Reference: ‘An extreme learning machine model for the simulation of monthly mean streamflow water level in eastern Queensland’, Environmental Monitoring and Assessment DOI 10.1007/s10661-016-5094-9