How good is my forecasting method? Some thoughts on forecast evaluation using cross-validation based on Australian experiences
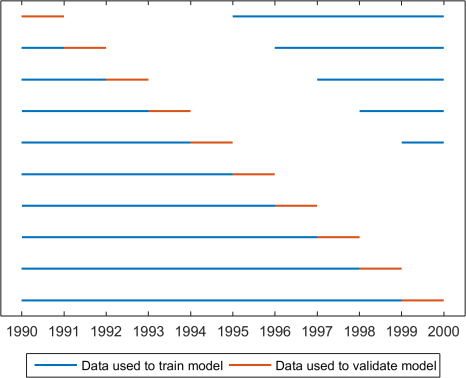
As hydrological forecasting researchers, we are often excited when we develop new methods that lead to forecasts with smaller errors and/or more reliable uncertainty estimates. So how do we know whether a new method truly improves forecast performance?
The true test of any forecasting method is, of course, how it performs for real-time applications. But this is often not possible, and we have to rely on assessing retrospective forecasts (hindcasts). One problem with assessing retrospective forecasts is that performance metrics can be overly flattering if care is not taken to address the so called ‘artificial skill’ problem.
Artificial skill is most prominent when the same set of data is used to develop forecasting models and to assess forecast performance. Cross-validation is one way to reduce artificial skill. Cross-validation is essentially the separation of model development and forecast evaluation. Forecast models are developed using one set of data and forecast performance is evaluated on another (independent) set.
Ideally, model development and forecasting in cross-validation will closely resemble the approach to be used in real-time. For real-time forecasting, it’s usual to calibrate models using as much data as is available before a forecast is issued. In cross-validation, we want to assess our forecasts on as many forecasts as possible across a wide range of conditions. Leave-one-out methods, shown graphically in Figure 1, are able to maximise the data available for training our models. As we (often!) have short data records, ‘leave-one-out’ methods of cross-validation instantly appeal.

Figure 1: A simple leave-one-out cross-validation scheme if we have data available for 1990-1999. Each line represents a different cross-validation run.
One problem with leave-one-out cross-validation schemes is that they allow us to build our model with data that are available after a forecast is issued. This is obviously not possible in real-time operations, and raises a thorny question: is it possible that data that occur after a forecast is issued can unfairly advantage our model?
For streamflow, the answer can be a resounding ‘yes’ (see Figure 2). Because catchments have memory, catchment conditions in say, 1995, can influence streamflows in 1996, 1997 or even longer. So if we are evaluating forecasts for 1995, we need to leave out not only data from 1995, but also 1996 (or more) when training our model.

Figure 2: Forecast errors in a catchment with long memory – the Harvey River in Western Australia. Error scores grow as more data are left out under the cross-validation.
The next question then is: how much data do I need to leave out? Our experience in seasonal forecasting is that we need to omit at least two years afterwards. In most cases, a ‘leave-5-years-out’ cross-validation is adequate, and we use this routinely when evaluating seasonal streamflow forecasts (Figure 3).

Figure 3: A leave-5-years-out scheme for streamflow forecasting. To account for catchment memory, data during and trailing each validation period are omitted.
What else has tripped us up?
- A perennial issue is the need to cross-validate all elements of forecasting system development. Many forecasting techniques select predictors (e.g. climate indices) that are then used in another model (e.g. a regression) to predict streamflow. In such cases predictor selection and model parameters usually need to be cross-validated to avoid overstating forecast performance.
- Forgetting to cross-validate reference forecasts can unfairly disadvantage your forecast method. Remembering to cross-validate the reference forecast (e.g. climatology) is just as important as cross-validating forecasts.
Depending on how you look at it, proper cross-validation of forecasts can be a bruising or enlightening experience, especially if a model performs fantastically in initial testing and then falters under cross-validation. Cross-validation is an essential component of every hydrological forecaster’s toolbox and ensures our forecasts stand up to the test of real-time.
And now, over to you:
- What are your experiences with cross-validation?
- Do you use different cross-validation schemes to those described here?
- Do you have any suggestions/methods for cross-validation that make the most of available data without unfairly advantaging your forecasts?
Original article posted on HEPEX, 8th March 2016 (Link)