Hydrologic similarity: Bridging the gap between hyper-resolution and hydrologic ensemble prediction
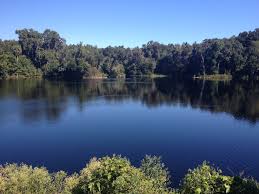
The ever-increasing volume of global environmental data and the continual increase in computational power continue to drive a push towards fully distributed modeling of the hydrologic cycle at hyper-resolutions (10-100 meters) [Wood et al., 2011]. In principle, this has the potential to increase model fidelity and lead to more locally-relevant hydrologic predictions (e.g., soil moisture at the farm level).
However, for the foreseeable future, due to computational constraints this modeling approach will not be suitable for large ensemble frameworks—a pre-requisite for reliable operational applications given the unavoidable uncertainties in model structure, model parameters, and meteorological forcing.
However, this does not mean that hydrologic ensemble prediction should continue to rely on over-simplistic hydrologic models simply to maintain computational efficiency. It is undeniable that providing field-scale hydrologic predictions can have the potential to significantly advance the use of hydrologic models (e.g., precision agriculture).
Furthermore, the important role of the physical environment and human management in hydrologic response necessitates a more explicit representation of the spatial drivers of heterogeneity in hydrologic models. Therefore, there is a need for a modeling approach that can provide field-scale predictions while approximating the computational efficiency of existing hydrologic models used in ensemble frameworks. Contemporary applications of hydrologic similarity can satisfy both objectives.
Hydrologic similarity
Hydrologic similarity aims to harness the observed covariance between a system’s physical environment (topography, soil, land cover, and climate) and its hydrologic response to assemble robust reduced-order models.
Although originally limited to one-dimensional binning of over-simplistic metrics of hydrologic response (e.g., topographic index), recent advances have taken hydrologic similarity a step further; a system’s most representative hydrologic response units (HRUs) are defined by clustering the high dimensional environmental data space [Newman et al., 2014]—the petabytes of readily available high resolution global environmental data make this feasible over the globe. Semi-distributed models can then be built to simulate these HRUs and their spatial interactions (e.g., HydroBlocks, Chaney et al., 2016).
Within the clustered spatial domain, each fine-scale grid cell (~30 meters) is associated with a specific HRU through its environmental characteristics. This then makes it possible to map out the HRU simulations onto the fine-scale grid to approximate the fully distributed simulation (see Figure 1 for an example).
Using this approach, ongoing work continues to show that the fully distributed simulation can be closely reproduced using around 1/1000 of the number of HRUs; each grid cell is a unique HRU in the fully distributed simulation. In other words, the semi-distributed model can effectively provide the same hydrologic information as the hyper-resolution fully distributed model with only a fraction of the computation.
This equates to being able to run roughly 1000 ensemble members of the semi-distributed model in the time it would take to run the fully distributed model once; all while being assured that each ensemble member closely approximates its corresponding fully distributed solution.
In summary, contemporary implementations of hydrologic similarity provide a unique opportunity to bridge the gap between physically-based hyper-resolution modeling efforts and hydrologic ensemble prediction. It enables robust ensemble frameworks to provide locally-relevant information while ensuring they can robustly characterize the unavoidable uncertainties due to model structure, model parameters, and meteorological forcing.
References
- Chaney, N., P. Metcalfe, and E. F. Wood (2016), HydroBlocks: A Field-scale Resolving Land Surface Model for Application Over Continental Extents, Hydrol. Process., doi:10.1002/hyp.10891.
- Newman, A. J., M. P. Clark, A. Winstral, D. Marks, and M. Seyfried (2014), The Use of Similarity Concepts to Represent Subgrid Variability in Land Surface Models: Case Study in a Snowmelt-Dominated Watershed, J. Hydrometeorol., 15, 1717–1738.
- Wood, E. F. et al. (2011), Hyperresolution global land surface modeling: Meeting a grand challenge for monitoring Earth’s terrestrial water, Water Resour. Res., 47(5).
Original article posted on HEPEX, August 15, 2017 (link)