Forecasting streamflow to 12 months – is it worthwhile?
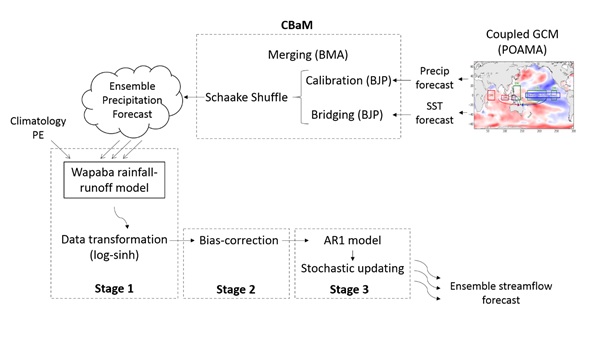
In 2010, the Bureau offered an operational seasonal streamflow forecast service to the public – probably the first such service in the world to be made publicly accessible. Users of the seasonal streamflow forecasts have provided positive feedback, but have asked for two additional features: i) that forecasts be broken down to 1-month streamflow totals and ii) that forecast lead times be extended. We have been working with the Bureau to try to produce forecast of monthly streamflow totals to 12-months ahead. Our recently published paper summarises the state of this research.
How do we predict streamflow 12 months in advance?
In our 12-month forecasting system, called FoGSS (Forecast Guided Stochastic Scenarios), we start with forecasts of rainfall from the POAMA (Predictive Ocean Atmosphere Model for Australia) climate prediction system. Direct outputs from climate prediction systems like POAMA are often not suitable for use in hydrological models. They tend to produce forecasts that systematically over- or under-state rainfall (in other words, they are biased), and they can make less accurate predictions than simply using historical climate (‘climatology’) as a forecast. To overcome these deficiencies, we use a statistical method called CBaM (Calibration, Bridging and Merging) to process rainfall forecasts over each catchment. We’ve discussed the benefit of calibration over simple bias-correction in a previous Ozewex blog post.
To forecast streamflow, we use the processed rainfall forecasts to drive simple conceptual hydrological models. For both rainfall and streamflow, the accuracy of the forecast comes from the initial conditions of the climate and catchment. POAMA assimilates climate and ocean observations before a forecast is issued, and then uses a complex computer model to evolve these conditions into the future. To understand how much moisture is in the catchment when our forecast, we drive our hydrological model with rainfall observations for 10 years up until the time the forecast is issued. The hydrological model then simulates the streamflow response to forecast rainfall.
Schematic of the FoGSS model
Dealing with the uncertainty of seasonal-annual prediction: generating an ensemble
Forecasts of climate or streamflow at seasonal time scales are inherently uncertain. Producing a range of possible outcomes – an ensemble – gives more accurate forecasts, on average, than using a single forecast. If the ensemble correctly represents the uncertainty in the forecast – the technical term is that the ensemble is reliable – it allows users to understand how uncertain forecasts are.
To generate an ensemble with FoGSS, we treat uncertainties from the rainfall predictions separately to those in the streamflow predictions. POAMA produces ensemble seasonal climate forecasts, but the ensemble tends to be overconfident (too narrow). So we use CBaM to calibrate the rainfall ensemble to make sure it is reliable. For streamflow, we devised a new error model to add uncertainty. The error model has three important jobs: first, it improves the streamflow predictions by looking at the most recent observations; second, it adds the correct amount of uncertainty for each month; and third, it propagates the uncertainty through the forecast.
Separating uncertainties from rainfall and streamflow predictions makes for a complex model, but it allows FoGSS to be highly modular. It means forecasters can, for example, swap in different hydrological models (we tried three) or use a different source of climate forecasts. The Bureau is currently upgrading its coupled climate forecast system from POAMA to ACCESS-S, so a modular approach ‘future-proofs’ the FoGSS system.
Did it work?
We tried FoGSS on 63 catchments, and for the large majority the system worked well. In general, forecasts were more accurate than climatology (historical streamflow) for the first 1-3 months, and after that the forecasts become like climatology forecasts. Forecast accuracy came mostly from catchment memory rather than from rainfall forecasts. In catchments with strong memory, forecasts could be informative to 6 months or more. However, forecasts were virtually never more accurate than climatology out to 12 months.
So why generate a 12-month forecast? In our discussions with water agencies, it’s clear that many rely on climatology to plan their operations. Because FoGSS forecasts transition to climatology, they are, at worst, similar to what water agencies are generally already using. When forecasts are very accurate, this information is passed on to the user; when forecasts are not accurate, forecasts are similar to what is already being used – all without requiring water agencies to chop and change datasets. In addition, it’s possible for users to sum forecasts from individual months to generate forecasts of, for example, 3-month total inflows. These ‘accumulated’ forecasts can cancel forecast errors from individual months (so an accumulated Jan-Jun forecast can be more accurate than climatology even if, say, the forecast for May was inaccurate), meaning that they are generally more accurate than climatology for 3-month total flows, and sometimes to very long accumulation periods. Overall, we think generating streamflow forecasts out to 12 months is worthwhile, provided users understand the limitations in these forecasts.
One type of catchment where FoGSS was not successful was in very dry, highly ephemeral catchments (catchments that flow less than half the time during dry seasons). In these catchments, FoGSS sometimes produced forecasts that were much less accurate than climatology (especially in months where the historical flow was always zero!). We are now working on ways to improve the streamflow error model to generate better predictions in ephemeral rivers.
Will 12-month streamflow forecasts be useful?
The hope for accurate, long-range streamflow forecasts is that they will allow water agencies to better manage our scarce water resources for agriculture, industry and the environment. We have shown in another paper that FoGSS forecasts generally improve the operation of reservoirs. Accurate long-range streamflow forecasts may allow us to spend less money on infrastructure (like very large reservoirs), they may help us to adapt to a changing climate and they may make our water trading markets more efficient. However, the use of seasonal streamflow forecasts is still in its infancy in Australia, and we don’t yet know whether these potential benefits can be realised. The Bureau is continuing to work with water agencies to explore the ways in which streamflow forecasts can be used to inform their operations – perhaps a worthy subject for a future blog post!
Article: “Assessment of an ensemble seasonal streamflow forecasting system for Australia. James C. Bennett, Quan J. Wang, David E. Robertson, Andrew Schepen, Ming Li, and Kelvin Michael, Journal of Hydrology and Earth System Sciences, 21, 6007-6030, 2017, https://doi.org/10.5194/hess-21-6007-2017“